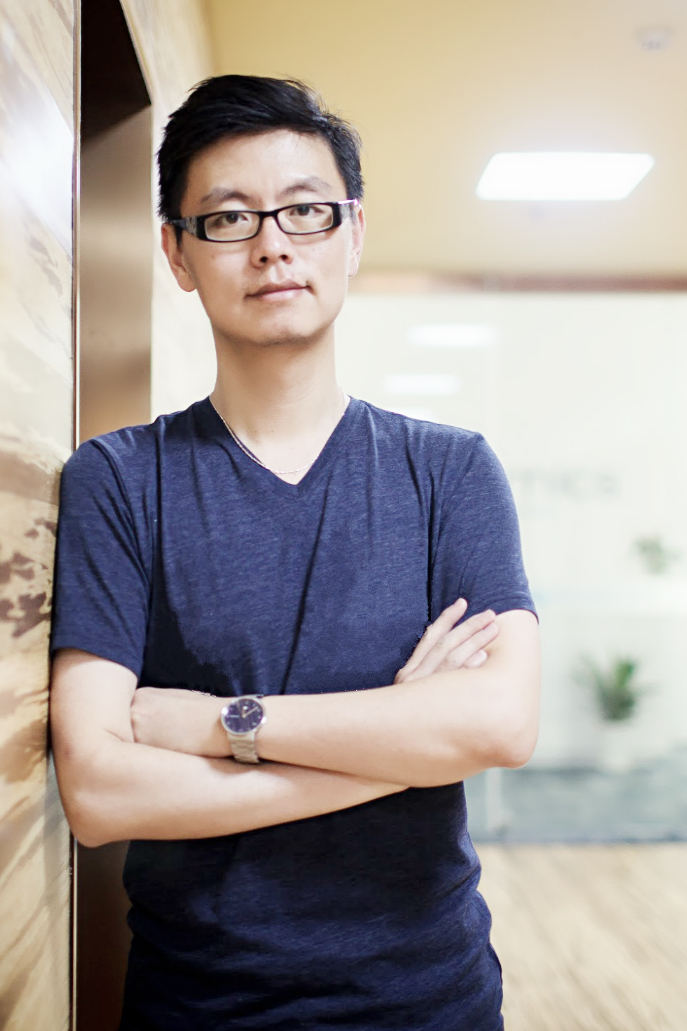
Jingjing Li, PhD
Health is a descriptive concept, which is often defined as “free from disease”. Our research aims to build a data-driven framework to quantitatively define health. We develop machine learning algorithms to define personal genome baselines for disease occurrences, and identify actionable lifestyle elements whose modification could compensate for genetic risk. We integrate personal genome baselines and individual lifestyles for precision health management.
Large-scale analysis of disease genomes
Because many complex human diseases have a strong genetic component, it has been long anticipated that once we can sequence one’s personal genome, we would be able to predict the person’s clinical outcome. This concept has been the cornerstone of today’s precision medicine. However, our genomic analysis of complex diseases is often challenged by mutational heterogeneity, where different patients often carry different sets of clinical mutations. We recently showed that these seemingly heterogeneous mutations are in fact convergent onto common pathways. We therefore use multi-omic profiling strategies to construct biological networks, and map genomic mutations onto biological networks to identify disease-associated pathways.
Clinical phenotyping using emerging technologies
In addition to using clinical records to model gene-environment interaction, we also leverage imaging and wearable sensor technologies to longitudinally digitize clinical traits. We use these technologies to derive quantitative traits for fine-mapping of genetic elements. We also perform digital signal processing to analyze these longitudinal data to capture subtle signals that will enable us to detect diseases before symptoms emerge. With comprehensively profiled individual lifestyles and physiologies, we aim to extend the classical P=G+E model to a Bayesian framework, where we stratify individuals based on personal genome baselines, followed by identifying lifestyle elements whose modification could improve clinical outcome.
Using iPSC-derived cells to identify pathogenic non-coding mutations
Our current understanding of disease genomes is largely limited to coding sequences, and the non-coding regions, accounting for ~98.5% of the human genome, have been largely unexplored for their contribution to disease etiologies. We perform ChIP-Seq and ATAC-Seq in iPSC-derived cells to delineate the genome-wide chromatin architecture in disease-related cell types, and build machine learning algorithms to identify non-coding mutations (SVs, indels and CNVs) that disrupt local and global chromatin architecture.